top of page
Published & Forthcoming Papers
Review of Financial Studies (2024)
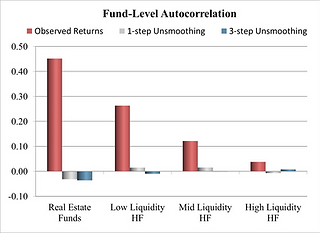

Abstract:
Funds that invest in illiquid assets report returns with spurious autocorrelation. Consequently, investors need to unsmooth returns when evaluating the risk exposures of these funds. We show that funds investing in similar assets have a common source of spurious autocorrelation, which is not addressed by commonly-used unsmoothing methods, leading to underestimation of systematic risk. To address this issue, we propose a generalization of these unsmoothing techniques and apply it to hedge funds and commercial real estate funds. Our empirical results indicate our method significantly improves the measurement of risk exposures and risk-adjusted performance, with stronger results for more illiquid funds.
The Journal of Real Estate Finance and Economics (2022)
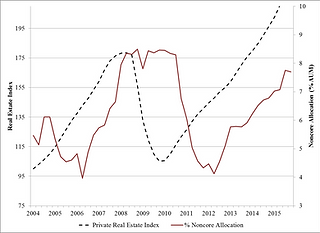

Abstract:
This paper documents that funds with greater non-core allocations have higher market risk exposure, β, but lower returns. Additionally, it documents that one reason their returns are lower is because they poorly time their investment into these properties. Open-end private real estate funds have higher non-core allocations at the top of the market and lower allocations at the bottom. As such, these funds are disproportionately exposed to the downside of the market. Lastly, I find that reaching for yield and fund flow pressure are important determinants of this phenomenon. Funds buy relatively more non-core properties when either the market return expectations or their net queues are smaller. Buying more core properties when queues are larger enables managers to place capital quicker, but it also hurts existing investors by decreasing their market risk exposure at the time when it is the most desirable and beneficial.
bottom of page